Cluster analysis in Python is a fundamental technique in data mining and machine learning used to identify groups or clusters within a dataset. It is widely applied in various domains, including marketing, biology, image processing, and customer segmentation. Python, with its rich ecosystem of libraries, provides powerful tools for performing cluster analysis efficiently and effectively. To begin with, we will discuss the essential concepts of cluster analysis. Clustering aims to group similar objects together based on their intrinsic characteristics and relationships. These clusters are formed in such a way that objects within the same cluster are more similar to each other than to those in other clusters. The choice of clustering algorithm and evaluation metrics depends on the nature of the data and the specific problem at hand.
Python offers several powerful libraries for cluster analysis, including scikit-learn, scipy, and K-means. Scikit-learn provides a comprehensive set of tools for machine learning, including various clustering algorithms such as K-means, DBSCAN, and hierarchical clustering. Scipy, a scientific computing library, offers functions for performing hierarchical clustering and distance calculations. K-means is a popular algorithm used for partitioning data into a predefined number of clusters. Read the following article curated trending cult to learn more about the best cluster analysis in Python, the best cluster Python course and the cluster Python course online.
What is Cluster Analysis?
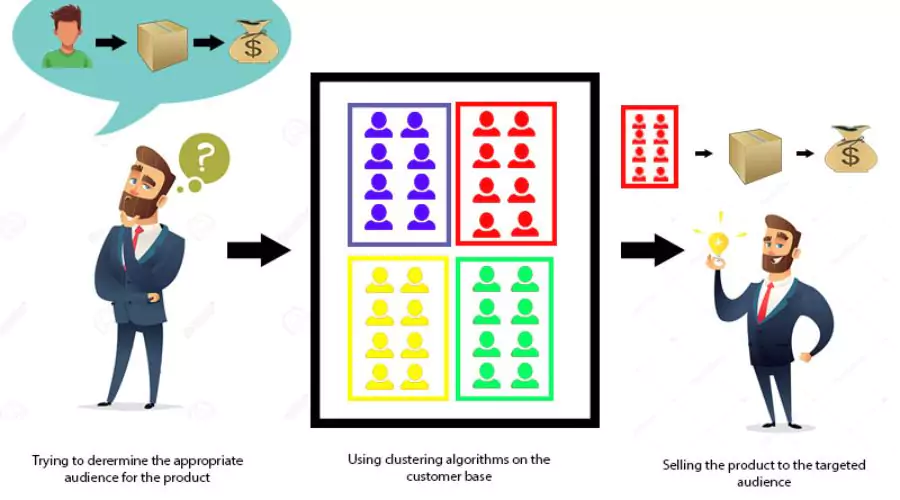
What is Cluster Analysis? | Neonpolice
Cluster analysis in Python is the process of dividing a dataset into groups, or clusters, based on the similarity or dissimilarity of the objects within it. The goal is to ensure that objects within the same cluster are more similar to each other than to those in other clusters. Cluster analysis has a wide range of applications, including customer segmentation, image processing, biological data analysis, and anomaly detection. To understand cluster analysis, it is important to be familiar with key concepts and terminology. We introduce terms such as clusters, distance metrics, and centroids. Distance metrics, such as Euclidean and Manhattan distance, measure the similarity between objects. The centroid represents the centre point of a cluster. Additionally, we discuss cluster validity and evaluation metrics to assess the quality of clustering results.
Preprocessing Data for Cluster Analysis
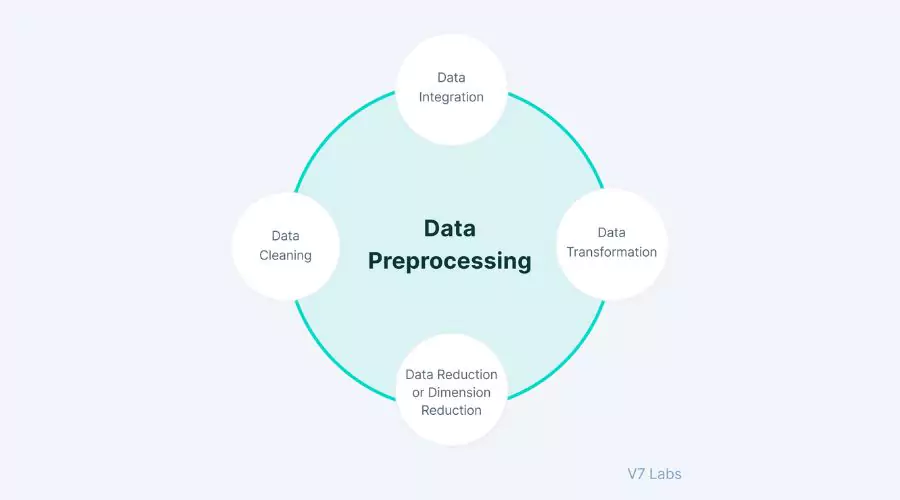
Preprocessing Data for Cluster Analysis | Neonpolice
Data preprocessing plays a crucial role in cluster analysis in Python. We delve into techniques for handling missing values, outliers, and categorical variables. These preprocessing steps ensure the data is in a suitable format for clustering. Feature selection is essential in cluster analysis to identify the most relevant features for clustering. We explore techniques like Principal Component Analysis (PCA) and t-SNE for dimensionality reduction, which can help visualize high-dimensional data and improve clustering performance.
Popular Clustering Algorithms and Implementations in Python
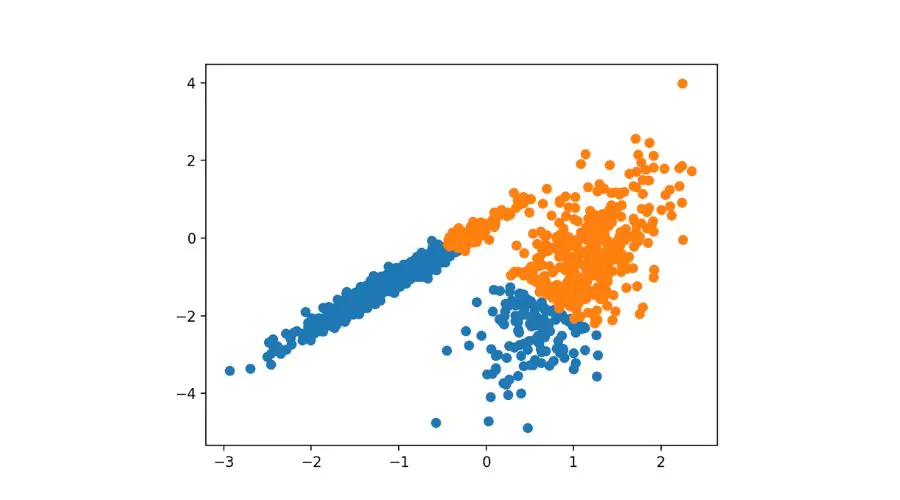
Popular Clustering Algorithms and Implementations in Python | Neonpolice
K-means Clustering
K-means clustering is one of the most widely used partitioning-based clustering algorithms. We explain the principles behind K-means and demonstrate its implementation using the scikit-learn library. We also discuss strategies for selecting the optimal number of clusters.
Hierarchical Clustering
Hierarchical clustering is a powerful algorithm that organizes data into a hierarchy of clusters. We explain the concepts of agglomerative and divisive hierarchical clustering and showcase their implementation with the scipy library. Dendrograms are introduced as visual representations of hierarchical clustering results.
Density-Based Clustering
Density-based clustering algorithms, such as DBSCAN, are suitable for discovering clusters of arbitrary shapes. We introduce the DBSCAN algorithm and demonstrate its implementation using scikit-learn. We also discuss how to interpret and evaluate DBSCAN results.
Internal Evaluation Metrics
Evaluating the quality of clustering results is crucial to assess the effectiveness of the algorithm. We explain internal evaluation metrics such as the Silhouette Coefficient and the Davies-Bouldin Index, which measure the cohesion and separation of clusters. We showcase their implementation in Python.
External Evaluation Metrics
In some cases, external evaluation metrics are used when ground truth labels are available. We introduce metrics such as Adjusted Rand Index (ARI) and Mutual Information (MI), which assess the agreement between the clustering results and the ground truth. We demonstrate the usage of external evaluation metrics in Python.
Conclusion
In this article, we have explored the world of cluster analysis in Python and its significance in uncovering patterns and structures within datasets. We started by understanding the core concepts of cluster analysis, including the definition of clusters, distance metrics, and centroids. We then delved into the preprocessing steps necessary to prepare the data for clusterings, such as handling missing values, outliers, and categorical variables, as well as feature selection and dimensionality reduction techniques. We explored popular clustering algorithms available in Python, including K-means, hierarchical clustering, and density-based clustering. Through practical examples and implementations using libraries such as scikit-learn and scipy, we learned how to apply these algorithms to our datasets and interpret the resulting clusters. We also discussed strategies for determining the optimal number of clusters and evaluated the quality of the clustering results using internal and external evaluation metrics. This is everything that you should know about cluster analysis in Python. Moreover, visit the official Trending cult website to learn more about cluster analysis in Python.
FAQs